Cognitive Science needs linguistic diversity
Unrepresentative samples can narrow our perspective.
There are roughly 7,000 languages around the world.1
Linguists divide these languages into 142 different language families, i.e., groups of languages all sharing a common ancestor. English, for example, is part of the Indo-European language family, which also includes languages like Bengali, Spanish, and about 442 other living languages.
Yet most research on language focuses on just a handful of languages. A 2020 survey of published Psycholinguistics articles found that 85% of them focused on just ten languages, dominated by English (which accounted for over 30% of these abstracts).
At least historically, the situation is even worse in computational linguistics. In a survey of papers published at various meetings of the Association for Computational Linguistics (ACL), the linguist Emily Bender found that anywhere from ~55% to 87% of these papers focused on English specifically. After English, the next-most common language was either German or Chinese (depending on the year), accounting for an additional 5%-23% of papers. Moreover, as Bender has pointed out, authors often fail to mention the language they’re studying when that language is English––English, all too often, is seen as the “default” language. She writes:
Work on languages other than English is often considered “language specific” and thus reviewed as less important than equivalent work on English. Reviewers for NLP conferences often mistake the state of the art on a given task as the state of the art for English on that task, and if a paper doesn’t compare to that then they can’t tell whether it’s “worthy”.[13] I believe that a key underlying factor here is the misconception that English is a sufficiently representative language and that therefore work on (just) English isn’t also language-specific.
In the study of human language, it’s clear why a biased sample is problematic. Different languages do things differently, but if you only ever look at English, you might mistakenly think other languages do things the same way.
For example, when describing events, English speakers use sentences like “the cat (S) chased (V) the mouse (O)”; thus, English is called a subject-verb-object (SVO) language. But many languages have different word orders. According to the World Atlas of Language Structures (WALS), which contains word order information for 1,376 languages, about ~41% of the world’s languages use a subject-object-verb (SOV) structure, and about ~14% lack a dominant word order entirely.
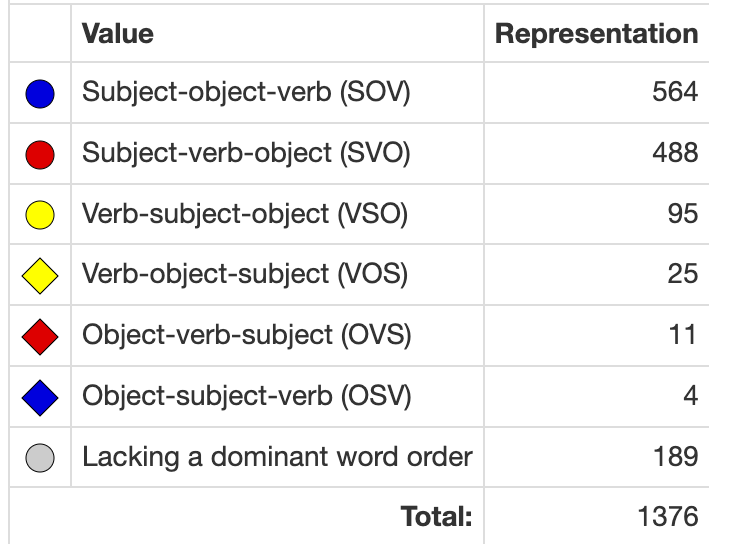
So people studying language should endeavor to study not just English but a diversity of human languages, lest they mistake the quirks of English for language “in general”. And fortunately, Linguistics has broadened its scope considerably––that’s precisely why resources like WALS can exist in the first place––even if there’s a long way to go.
A stronger claim, however, is that this is problematic not only for linguists but for cognitive scientists more generally––that an over-reliance on English has hindered our ability to understand the human mind in all its complexity. That’s the claim advanced in a 2022 article by Damián Blasi and others, and that’s what this post will focus on.
Why do samples need to be representative?
Scientists are typically interested in generalizations––statements that apply to large swaths of the population of interest (whether that be brains, societies, or languages).
But studying the entire population isn’t ever possible. Even if you could somehow manage to survey every human in the United States––something even the census struggles with––you’re limiting your analysis to one country with a very particular culture. And even if you managed to also survey every human in the world, no matter where they lived, your analysis would be limited to a very particular slice of time: mostly industrialized societies in the 21st century.
So we have to rely on the logic of sampling: we carve out a slice of the population we’re interested in, and try to generalize from this sample to the larger pie. But in order for this generalization to work, the sample needs to be representative: it should accurately reflect the characteristics of the broader group.
Unfortunately, research on human behavior––and humans more generally––is often conducted on unrepresentative samples. For example, most behavioral psychology research has historically been conducted on undergraduates at four-year research universities––a population that likely diverges from the broader category of “human beings” in all sorts of ways. This is why Joe Heinrich and others coined the term “WEIRD” back in 2008, meaning: Western, Educated, Industrialized, Rich, and Democratic.
The point of this is not to throw stones at psychologists (or linguists, for that matter). There are understandable reasons why researchers would rely on what’s essentially a convenience sample: for one, it’s very hard to recruit participants from populations around the world, and there’s already a ready-made pool of candidates in the undergraduate population. And historically, much of this research has of course occurred at universities, and many of those universities are located in Western countries that are rich enough to fund the research. To their credit, psychologists take this problem seriously and are trying to expand their samples.
The interesting claim made by Blasi et al. (2022) goes beyond the observation of “WEIRD” subjects, however: it’s that an over-reliance on English specifically––in terms of the research subject, in terms of the lingua franca of academic publishing, in terms of the language spoken by the researchers themselves––has led to a narrow view of the human mind.
How English limits our perspective.
English is different from lots of other languages in lots of different ways. Importantly, these differences have “knock-on” consequences (or at least correlates) for other domains of cognition––such as perception, memory, and even social interaction.
English is written, and it’s written in a particular way.
For one, English has a widely used writing system. Many languages (about 40%) do not have a writing system. This is a hard topic to study, but some psycholinguists have theorized that writing shapes our metalinguistic awareness, i.e., our internal understanding of how language works. Even the idea of what constitutes a “word” or “sentence” could plausibly be affected by formal training in reading and writing.
Even among languages with writing systems, there’s considerable diversity. English uses an alphabetic writing system, but many languages learn non-alphabetic scripts, e.g., abjads (where only consonants are represented) or morpho-syllabaries (where characters stand for entire syllables).
Yet according to a recent survey, most research (~57%) on reading uses English, and thus the English writing system, as its domain of study.
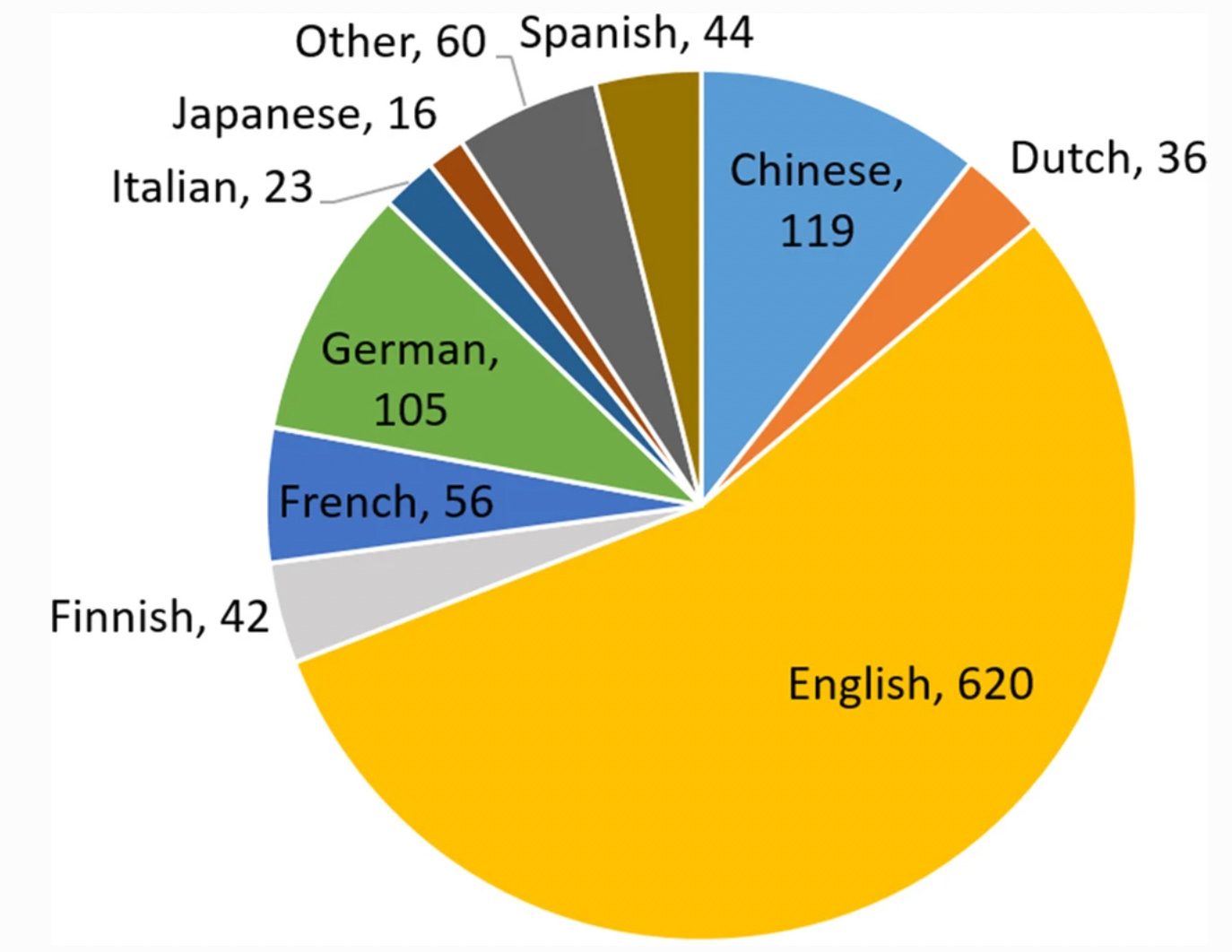
Further, English also has a specific writing direction (left to right). There’s now considerable evidence that writing direction is correlated with the spatial metaphors one uses for time. In experimental tasks, English speakers tend to place early events on the left and later events on the right; speakers of Hebrew, which is written right-to-left do the opposite. In a similar task, Chinese speakers sometimes placed events left to right, and sometimes top to bottom––with the latter tendency reflecting the top-down writing direction of Chinese.
English vocabulary carves up the world in a particular way.
Different languages also carve up the world in very different ways.
This is particularly obvious in the domain of color. In some cases, English is missing lexical distinctions that other languages routinely make, e.g., Russian distinguishes between different shades of blue. In other cases, English makes a distinction that other languages don’t, e.g., so-called “grue” languages use the same word for green and blue. There’s evidence that these distinctions aren’t purely linguistic––that they show up in signatures of neural processing as early as 100ms after viewing a color.
Languages also vary in which domains they deem most important. Aristotle famously proposed a “universal hierarchy” of the senses, from vision (most important) to taste (least important). And indeed, visual concepts are over-represented in the vocabulary of many languages (such as English); English speakers also seem to agree more, i.e., use the same term, when describing visual features than features like smell or taste.
Yet this pattern––contrary to Aristotle’s claims––is by no means universal. In a survey of 20 typologically diverse languages (including 3 sign languages), Majid et al. (2018) found surprising variation in which sensory domains were most codable. While languages like English followed (roughly) Aristotle’s presumed hierarchy, languages like Tzeltal and Umpila showed very different patterns: for example, the most codable sense in Tzeltal was taste, while the most codable sense in Umpila was smell.
Some of these differences are strongly related to the composition of a language community. Terms for smell are much more codable in languages spoken by hunter-gatherer communities than languages spoken in industrialized societies––hearkening back to the “I” in Heinrich’s “WEIRD” acronym.
English is spoken.
There are at least ~300 signed languages used around the world. Some of these languages have fascinating origin stories, such as Nicaraguan Sign Language (NSL), which developed spontaneously when a school in Nicaragua attempted to teach Spanish to deaf children who’d previously not learned a sign language.
Recently, evidence has been mounting that language modality has impacts on cognition more broadly. In particular, there seems to be a trade-off in terms of which components of working memory are strengthened or recruited more frequently: signers display reduced verbal working memory (relative to users of a spoken language), but enhanced spatial working memory (again, relative to users of a spoken language), as well as enhanced mental rotation abilities.
These differences never would’ve been discovered if cognitive scientists had not expanded their domain of inquiry to signed languages, overturning preconceived notions about which cognitive capacities were fixed and universal and which were subject to variation.
English has a fixed word order.
As I mentioned above, English has a particular word order: subject-verb-object.
But many languages don’t require speakers to describe participants in an event in a fixed order; these languages typically rely on other grammatical mechanisms, such as case marking, to indicate “who did what to whom”.
This fact has interesting implications for both the human mind and the success (or lack thereof) of Large Language Models (LLMs). One popular theory of language processing posits that humans predict upcoming words using information about which words came before––this falls under the broader category of “predictive coding”, which postulates that the human brain evolved to make accurate predictions about the environment. Similarly, as I’ve written about before, LLMs are trained a next-word prediction task requiring them to form a probability distribution over upcoming words, given previous words in the sentence. Some researchers have even claimed that the success of LLMs––both as models of language, and as models of human language processing––provides evidence for the viability of a next-token prediction mechanism.2
Yet most research on predictive coding––and most LLMs, for that matter––focuses on languages like English, which have fixed word order. How exactly does predictive coding work when applied to languages without fixed word order? Presumably, it would not be particularly efficient for the brain to predict upcoming lexical tokens, given that a speaker has so many degrees of freedom in terms of which token they use. This is not to say that no prediction of any kind occurs––certainly, comprehenders likely predict or at least “fill in” semantic features of the event being described––only that the story is more complicated when it comes to next-token prediction specifically.
Moreover, because LLMs are primarily trained and tested on languages with fixed order (like English), we simply don’t know as much about how these principles translate to other languages without fixed word order.
We do know, however, that one underlying assumption of much research on LLMs––that bigger is better––isn’t always true. In English, larger LLMs tend to have lower “perplexity”, meaning they are better at predicting upcoming words; they’re also better at predicting the time that English speakers spend reading different sentences. But this isn’t true in Japanese: larger models do have lower perplexity, but they’re no better on average at predicting how long Japanese speakers spend reading different sentences. This particular result isn’t necessarily due to “fixed” vs. “free” word order––Japanese does have word order (SOV)––but it does suggest that we should be cautious about drawing conclusions from research that’s been conducted overwhelmingly on English-speaking populations, with models trained primarily on English.
Other cross-linguistic differences abound.
The article discusses many other examples of how an over-reliance on English has led Cognitive Sciences to make premature generalizations on human cognition more generally.
To name just a few others:
Some languages encourage “redundant” or repetitive back-channels (e.g., repeating what someone’s said rather than saying “uh-huh”), seeming to violate the presumed drive for conversational efficiency across languages.
Some languages (unlike English) have different words denoting beliefs that are true vs. beliefs that are false; and speakers of these languages also display better performance on tasks measuring Theory of Mind––the ability to reason about mental states.
The syntactic structure of your language is correlated with whether you’re better at remembering the first items on a list or the last items on a list.
Moving past the “original sin” of Cognitive Science.
To sum up: Cognitive Science aims to be the interdisciplinary study of the human mind in general, yet most research has focused on the minds of English speakers in particular.
This is a problem not only for theories of language, but for theories of cognition more generally. The language you speak, after all, has all sorts of knock-on consequences (or correlates) with other aspects of cognition––potentially including your meta-linguistic awareness, your working memory, and which sensory modalities you prioritize.
As with any issue, there are two necessary components to addressing this challenge:
Recognizing the problem.
Doing something about it.
I’m actually pretty optimistic about (1). In my experience, many Cognitive Science practitioners agree that Cognitive Science would benefit greatly from incorporating more linguistic diversity––including not only speakers of different languages but also speakers of multiple languages, as well as sociolinguistic variation.
Of course, talk is cheap. It’s easier to say that one “recognizes” the importance of linguistic diversity than to actually do something about it. Still, I think it’s good to acknowledge that progress has been made on this front––and that there are worse counterfactuals to consider than the present moment (e.g., the state of the field circa 50 years ago, or even ten years ago).
This leaves (2): what can (and should) be done?
A systemic problem isn’t going to be rectified overnight––it requires buy-in at both the individual and institutional level. And in the latter case, true change will likely require considerable changes to the flow of capital through academic institutions, granting agencies, and publishing houses. The authors point out:
It is hard to envision a radical change in the field if institutions (universities, journals, funding bodies) do not commit to research that seeks to systematically explore, generalize, and falsify our models of human cognition by exploring non-English-speaking peoples and societies.
With these kinds of sweeping changes, it’s hard to know where to start, but I can certainly list things I’d like to see.
For example, I think it’d be great if granting agencies (e.g., the NSF) explicitly committed more resources towards improving linguistic diversity in Cognitive Science research––this could include documentation of under-resourced languages, recruiting historically under-represented participants for psycholinguistic experiments, and even building language technologies for low-resource languages. It’s important to note that much of this work is already being done: here at UCSD, for example, Ndapa Nakashole has worked on expanding English-based language technologies to low-resourced languages such as the Bantu languages, which are spoken by over 350 million people. What I’m arguing is that granting agencies need to incentivize even more researchers to do this work, and of course reward the researchers who already are doing it.3
Academic publishers could also play a huge role, e.g., by hiring professional translators to ensure that an individual’s native language is no longer a barrier to getting published––or to reading the published work that’s already out there. Getting publishers to actually do this is a separate challenge. But more papers than ever are being circulated these days on arXiv and other preprint servers––so if academic publishers want to stay competitive, they should offer services that universities and individual researchers will value.4
The authors also suggest some steps that individuals can take:
Individuals studying language should consult (and ideally help contribute to) growing databases that document linguistic diversity, such as WALS.
Individuals recruiting human subjects should take advantage of web-based recruiting platforms to broaden their participant pool.
Individuals can conduct (or collaborate with individuals conducting) cross-cultural and cross-linguistic studies.
Individuals can establish ties between institutions within the Anglosphere to those outside it.
The authors themselves are a good example of following these guidelines. Asifa Majid, for example, has conducted extensive cross-linguistic and cross-cultural research on the language of olfactory perception, including research involving hunter-gatherer groups like the Jahai. Damián Blasi, another co-author, has published work on sound symbolism––the phenomenon whereby words sound like what they mean––that includes analyses of thousands of extant languages.
One other suggestion I’d add at the individual level is incorporating all this research into pedagogy. This is less about impacting the sphere of published work, and more about influencing the next generation of cognitive scientists and what they consider the purview of their field.
If your undergraduate (and graduate) training only involves research conducted by and on English speakers, then the Cognitive Science you go on to practice will likely be the same. But if your education involves examples highlighting the remarkably diverse ways that humans across the world communicate, then you’ll likely bring that lens to bear on any work you do––both within and beyond the field.
It’ll require some updating of old syllabi, but I think it’s worth it.
Exact estimates vary, depending on exactly how one defines a “language” and also what time sample one is drawing from. Many languages spoken in the past are now extinct, and many current languages (~40%) are unfortunately endangered.
This is itself a controversial or at least complicated claim. As a recent paper argues, the success of a next-token prediction mechanism in explaining human brain activity does not demonstrate that human brain activity operates according to predictive coding––rather, next-token prediction is one learning objective (among many) that leads LLMs to extract useful features about language, which can therefore be used to predict how the human brain responds to linguistic input. It’s a subtle but important distinction.
Which is not to say no work on linguistic diversity gets funded! This 2021 award, for example, committed almost $3M to adapting linguistic technologies to regional varieties of English.
Many multilingual researchers already do freelance work translating academic articles on the side; it’d be nice to institutionalize these positions so those individuals can get paid better for their labor.