What "language" is a language model a model of?
The sociolinguistics of large language models.
Large Language Models (LLMs) like ChatGPT are computational systems trained to predict the next word (or “token”) in a sequence of words. This training process—along with other accoutrements, such as reinforcement learning with human feedback—has produced systems that display surprisingly impressive behavior. Yet for all the excitement and debate about what these systems can and can’t do, there’s surprisingly little discussion about the details of that second “L” in “LLM”: language.
LLMs are trained on a corpus of text. But which language, from which language family? What register and dialect of that language? From which time period? As I’ve written before, LLM training data is a biased sample, and this has implications for the behaviors we observe in the LLMs on offer. One answer to this is to suggest we train systems on more representative training data. But “representative” of what? There are so many varieties of even a single language that it may not always seem sensible to draw generalizations across them.
A recent arXiv preprint by Jack Grieve and nine other co-authors entitled “The Sociolinguistic Foundations of Language Modeling” argues that LLMs are models of a specific variety of language, and that this fact tends to be neglected in discourse around LLMs. The authors point out some of the problems this leads to, and also make some concrete suggestions—both in terms of how we select our training corpora, and in how we talk about the LLMs we do train.
What is a language “variety”?
A “variety” is a specific type of a language, defined by specifying the circumstances under which language is produced. It’s a term from Linguistics, where researchers generally try to be as precise as possible about which collection of linguistic practices they’re characterizing. As the authors write (bolding mine):
One reason a variety of language is such a powerful concept is because it can be used to identify a wide range of phenomena – from very broadly defined varieties like the entire English language to very narrowly defined varieties like the speeches of a single politician…Although what are traditionally considered entire languages like English or Chinese can be referred to as varieties, the term is most commonly used in linguistics to refer to more narrowly defined types of language. (Pg. 3).
That is, the term can in principle refer to quite broad categories (“English”), but in practice, it’s used to carve out something more specific. Linguists have all sorts of words for narrowing down what they mean by “something more specific”, which again range in generality—from dialect to idiolect. Here, the authors suggest it is useful to taxonomize varieties according to three sources of variation.
First, a dialect is a variety “defined by the social backgrounds and identities of the people who produce language” (pg. 3). In other words, the term “dialect” encompasses social characteristics such as nationality, region, class, or ethnicity.
Second, a register is “defined by the social contexts in which people, potentially from any social background, produce language” (pg. 3). For example, a late-night conversation with a friend is a very different social context than a formal speech by a politician.
Third, a period is “defined by the time span over which language is produced” (pg. 3). Languages aren’t static—so the English of the 19th century isn’t exactly the same as the English of the 21st century.
Again, the term is quite flexible, which is part of its utility. Regardless of whether we’re referring to “American English” or “the English I use in my Substack posts”, we can characterize both those varieties of English using the three sources of variation above: dialect, register, and period.
The authors also include a very helpful figure that illustrates the hierarchical nature of language varieties. Professor Jack Grieve, the first author of the paper, graciously allowed me to include the figure in this post. As the figure shows, varieties “vary” along at least three dimensions, and each of these dimensions can be made more and more specific. The sub-variety “contemporary instruction” can be further decomposed into “online lectures” and “in-person lectures”, while “face-to-face dialogue” can be broken down into “dinner conversation” and “late-night conversations”.
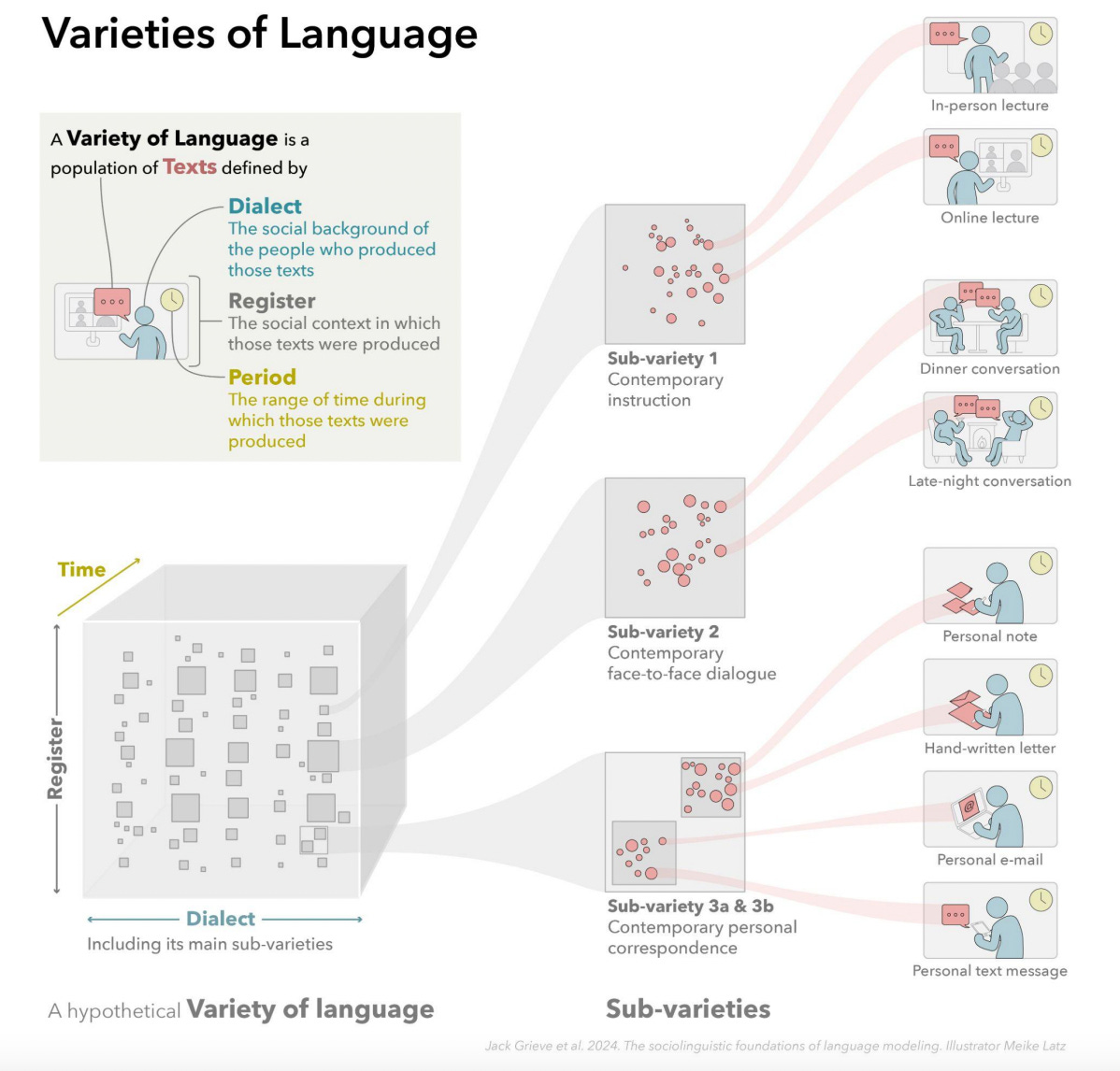
In turn, linguists interested in characterizing a variety will try to gather data representative of that variety. Depending on the variety in question, this might include spoken or signed utterances, longform journalism, lecture transcripts, text message data, and more. The linguistic data used for this kind of research is called a corpus.
The importance of representativeness
When making claims about a language or language variety, it’s very important that the corpus being used to draw generalizations is actually representative of the thing being studied. This is hard to do—you can’t just download a text dump of the Internet and call it a day.
And you also can’t escape this challenge by just asserting that you’re not interested in a specific language variety. As the authors write:
It is also important to stress, especially in the context of language modeling, that any corpus – any sample of texts – inherently represents some variety of language, namely, the smallest common variety that encompasses that sample of texts. (Pg. 6).
In other words, you’re working with language varieties whether you know it or not. If we’re not careful about specifying what variety we’re interested in—the set of dialects, time periods, and registers we want to study or model—then the corpus we build will likely reflect a convenience sample (whatever was available at the time), and we simply won’t know what cross-section of sub-varieties we’ve included. In contrast, if we define what variety we want to characterize, then we can curate a corpus of texts. Again, this is hard, but the pay-off is worth it: we can be more confident about what we’re actually making claims about.
What does this mean for language models?
Language models are trained on a (typically quite large) corpus of text. Researchers and companies building language models rarely specify the sociolinguistic details of that corpus, but it nonetheless represents a particular sample of all the things that could be produced in language.
Grieve et al. (2024) argue:
Our primary contention in this paper is that language models, which are trained on large corpora of natural language, are therefore inherently modeling varieties of language. In other words, we conceive of language models as models of language use – models of how language is used to create texts in the variety of language that the corpus used to train the model represents. (Pg. 6).
This isn’t usually how we talk about large language models. First, even the primary “language” an LLM is trained on (or tested in) isn’t always specified when researchers or companies make claims about what “LLMs” can and can’t do, or about an important research result obtained using LLMs. Is it a model trained on English? Spanish? Arabic? What language was the assessment written in?
Second, and more to the point: even if the language is specified, the language variety typically is not. A training corpus necessarily represents a collection of language uses, and those uses can be characterized in terms of dialect, register, and period. For example, perhaps a corpus used to train an English LLM consists of 60% Wikipedia articles (contemporary written online text with a pedagogical purpose), 20% news articles dating back to 1987 (written journalism from the last few decades), and 20% social media posts1 (contemporary written online text produced primarily for entertainment and/or social connection). That’s distinct from a corpus where those ratios are different, or a corpus composed primarily of text produced during the Victorian Era., or a corpus of transcripts of late-night phone conversations.
In terms of why it matters, the authors argue:
we believe that many problems that arise in language modeling result from a mismatch between the variety of language that language models are effectively intended to represent and the variety of language that is actually represented by the training corpora. (Pg. 6).
The challenges ahead
The authors suggest that language models trained on unrepresentative corpora—whose sociolinguistic details may not even be known to the researchers building the model—can lead to a couple problems. They also suggest some possible paths forward, which may address those problems and also contextualize other findings with respect to LMs.
One concrete problem is social bias, i.e., an outcome that unfairly disadvantages or harms certain groups. In the case of LLMs, this could manifest as harmful stereotypes (e.g., if the training data contains disparaging viewpoints about certain social groups), or simply as worse quality of service (e.g., with users from a language variety that’s underrepresented in the training corpus). The latter case is particularly clear if we consider the extreme case of entirely different languages (e.g., English vs. Spanish). British English and American English are obviously much more similar than English and Spanish, but there are notable lexical differences: an LLM trained on only American English text would be less likely to correctly understand words like “boot” (for trunk) or “underground” (for subway).
A second challenge is language change. Languages aren’t fixed—they change over time, reflecting changes in culture, as well as contact with other languages and cultures. Even ignoring the other sociolinguistic dimensions of register or specific dialect, this means that an LLM trained on English of the 19th century will be, to some extent, “out of step” with modern English speakers. Thus, LLMs will likely need to be retrained (or at least fine-tuned) on up-to-date corpora. And, of course, widespread usage of LLMs might mean that the modern Internet will consist of more and more LLM-generated text. The authors suggest that LLM-generated text is perhaps best characterized as another “register” of language use, and as I’ve written before, this could even change how humans use language. From this perspective, including some LLM-generated text in an updated training corpus might be appropriate, but only if it’s very clear that this does represent, in fact, a new register.
This potential solution of fine-tuning LLMs to keep them up-to-date can be situated in the language of domain adaptation. LLMs often benefit from further fine-tuning (e.g., for a chat interface); perhaps fine-tuning across time is the right way to think about accounting for the fact that languages change. Similarly, fine-tuning might be employed to address issues of underrepresentation. The authors suggest that a “base LLM” could be fine-tuned (i.e., adapted) using a more carefully curated corpus intended to represent a target language variety or varieties. The corpus itself still needs to be built, of course—which is no small feat—but conceptually, it makes sense to think of this as “honing” the base LLM to a more specific, well-defined variety of language. Whether that’s feasible will probably depend on the quality of the new corpus, as well as the relationship between the varieties present in the original corpus and those in the new one.
Finally, the authors connect this idea to research on model scale. There’s a line of research that suggests bigger LLMs—more parameters and more training data—are generally better at predicting the next word, and also often (but not always) at downstream tasks. Yet a larger training corpus doesn’t necessarily mean a more representative one, as Emily Bender and others have pointed out. Here, the authors suggest that research might benefit from experimenting not only with the scale of training data but also its diversity. A wider exposure to more varieties of language patterns might improve the ability of a model to make predictions—certainly when it comes to language varieties that are traditionally under-represented in a model’s corpus.
What are we modeling, anyway?
A “language model” is just a system that assigns probabilities to sequences of words; typically these probabilities are learned by “training” that system on lots of language data. But what exactly is this a “model” of?
For many people excited about LLMs, the “language modeling” component might feel like a means to an end. The supposition is that training a neural network on lots of language leads to the kind of system that will eventually display intelligent behavior—perhaps because learning to predict words requires learning things about the world or even the people producing those words.
But these systems are, at the end of the day, models of language—and not just “capital-L Language”: they’re models of a language variety or collection of varieties. As Emily Bender has pointed out, researchers in this space don’t always bother to point out which language they’re studying, with English as the unstated “default language”. I’m generally in support of researchers being more precise about what they’ve done and what they’re claiming, so I’m fully in support of more care and attention paid to the question of not only what language we’re modeling but also what language variety. This will require doing work that’s not as flashy as building a new system—e.g., creating, curating, and describing text data—but it’s important work to be done.
Related posts:
One might fruitfully decompose “social media” into different platforms or sub-communities as well, to the extent that this provides information about the language variety or varieties being studied.
Great post, Sean. This explanation of variations in what Saussure called “parole,” language in use, vs “langue,” language as an object, refocuses the question of what LLMs are actually doing and why they can seem weird. Is it reasonable to say that the LLMs software is built around language as object but the training derives from language in use? Chomsky’s genius was to explore a grammar of language in use, which is the basis of computational language. This whole question of sociolinguistics and the bot is fascinating.