I’ll start with a question: How many animals of each kind did Moses take on the ark?
Many people will give the answer “two”. But of course, the biblical story of the flood and the ark doesn’t feature Moses—it’s about Noah. In a now-famous 1981 study, more than 50% of participants who knew the correct answer (i.e., they were familiar with the Bible and the story of Noah’s ark) failed to notice the incongruity in this question, almost as if they automatically corrected “Moses” to “Noah” in their head while reading. In fact, when they were asked to repeat the sentence later in the experiment, 25% of them did explicitly make this correction.
This is an example of what’s called a semantic illusion. Semantic illusions occur when comprehenders fail to notice inaccuracies or inconsistencies in language. And they’re not just limited to Biblical stories. Consider the questions below:
What did Goldie-Locks eat at the Three Little Pigs’ house?
What country was Margaret Thatcher president of?
Can a man marry his widow’s sister?
As with the “Moses illusion” above, many people fail to notice the incongruities in these sentences.1 It’s almost like they’re forming a rapid “guess” about what the question is asking—based on somewhat superficial word associations—and jumping to what they take to be the right answer.
There’s now a wealth of research building on this initial finding, exploring the conditions under which people do or don’t notice these incongruities. One factor is the semantic relatedness of the “distractor” word (e.g., “Nixon” vs. “Moses”), as is depth of processing (e.g., whether it’s written in hard-to-read font that encourages a closer read).
Most importantly, semantic illusions are one piece of evidence in a broader research program that suggests humans don’t always process language all that deeply—often, we rely on “good-enough” representations that allow us to get by (most of the time!).
The old view: “deep” understanding
The importance of this new “good-enough” view can be best understood by comparing it to the old view.
Historically, language comprehension was viewed as a “deep” process, whereby comprehenders built a fully compositional representation of a sentence and derived the meaning of that sentence from the sum of its parts. This view is closely associated with linguists like Noam Chomsky, and there are a few important assumptions built in.
The first assumption is that language processing involves building a hierarchical “parse” of a sentence’s structure, often expressed as a tree (see below). For example, a sentence like “The dog chased the mouse” would be recursively parsed into its constituent parts, mapping from each of the “leaf nodes” (the individual words) up different “branches” of the tree until a complete sentence representation is formed. There are lots of debates about how this parsing happens or exactly what its structure should be, but the consensus is that something like it does indeed happen.
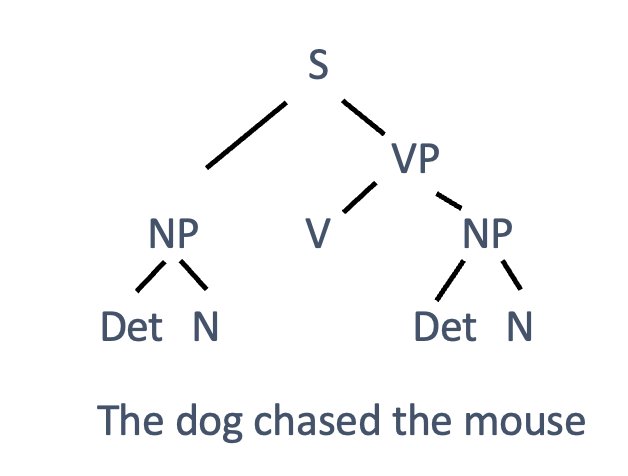
A second assumption, which follows from the first, is that no word can be part of multiple “branches” of this tree. For example, the word “dog” couldn’t be part of the subject noun phrase (NP) and part of the verb phrase (VP).
And a third assumption—emphasized by some but not shared by all—is that different surface structures nonetheless point to the same “deep structure”. That is, the active sentence above (“The dog chased the mouse”) has the same deep structure as its passive alternation (“The mouse was chased by the dog”).
There’s a lot to like about this view. It’s clean and elegant, and has deep connections to theories of formal logic and data structures (e.g., a binary search tree). Most importantly, it captures the intuition that human language has hierarchical structure.
The question is whether and to what extent humans routinely parse language in exactly this way.
The “good-enough” view
An alternative view is that people often rely on relatively superficial “parses” of the sentences they encounter. Language, after all, unfolds quite quickly, and turn-taking happens fast (~200ms on average). Moreover, language is often fragmentary, full of disfluencies (“uh” and “um”)—far cry from the perfectly grammatical exemplars used to demonstrate hierarchical parsing. It makes sense, then, that people would need to rely on shortcuts to rapidly determine what something means. This view is sometimes called “good-enough comprehension” and is closely associated with researchers like Fernanda Ferreira.
There are a few key pieces of evidence to support this view. The first is the existence of semantic illusions, as I discussed above. Presumably, if people were building truly deep representations of language, they would notice semantic inconsistencies like “Moses”. Instead, it seems as though at least part of language comprehension involves a kind of pattern-matching procedure. (Something like: “Moses” is associated with the Bible, as is “the ark”, and the story of the ark involved two animals of each kind, so the answer is “two”.)
The second is evidence for what’s sometimes called “shallow parsing”, which violates those assumptions above hierarchical parsing I mentioned earlier. Consider the sentence:
While Anna bathed the baby played in the crib.
Now I’ll ask you two questions:
Did the baby play in the crib?
Did Anna bathe the baby?
Grammatically, the sentence should be parsed like “[While Anna bathed] [the baby played in the crib]”. That is, Anna is the subject of the “bathing” event, and the baby is the subject of the “playing in the crib” event. Based on this understanding, the correct answer to the first question is “yes”, and the correct answer to the second question is “no”.
But that’s not what everyone answers. Specifically, most people (near ~100%) answer “yes” to the first question, but many (~30%) also answer “yes” to the second question. The logic kind of makes sense: when you first read the sentence, you might have been led down the garden-path and interpreted the first verb (“bathed”) as a transitive verb relating Anna to the baby (“While Anna bathed the baby…”). This is a well-known effect in psycholinguistics, and is induced by a kind of transient ambiguity in how to interpret the structure of a sentence.
But what’s most notable here is despite (presumably) adjusting their interpretation to process the words that follow, many people fail to reconcile these conflicting interpretations. That is, they interpret “the baby” both as the object of bathing and as the subject of playing. Grammatically, however, this should be impossible! That would imply that “the baby” is a member of multiple branches of the same tree—which violates a key assumption of fully compositional parsing.
This pattern can be contrasted with people’s responses when given a sentence like:
The baby played in the crib while Anna bathed.
In this case, 100% of participants correctly respond “yes” to the question about the baby playing in the crib, and approximately 0% of participants incorrectly respond “yes” to the question about Anna bathing the baby. That’s because this sentence doesn’t induce a temporary ambiguity—so there’s no garden paths to reconcile.
Another interesting finding relates to that third assumption we discussed earlier: that active sentences (e.g., “The dog bit the man”) are translated to the same deep structure as passive sentences (e.g., “The man was bitten by the dog”). A 2000 paper (Ferreira & Stacy, 2000) presented participants with sentences like:
(1) The man bit the dog [Active + Implausible]
(2) The man was bitten by the dog [Passive + Plausible]
(3) The dog bit the man [Active + Plausible]
(4) The dog was bitten by the man [Passive + Implausible]
For each sentence, participants were asked to indicate whether the event described in the sentence was plausible or implausible. For sentences like (1), (2), or (3), most participants answered correctly: implausible sentences (e.g., “The man bit the dog”) were classified as implausible, and plausible sentences (e.g., “The dog bit the man”) were classified as plausible. But implausible sentences in a passive form (e.g., “The dog was bitten by the man”) were more likely to be answered incorrectly.
One way to think about this is that people rely on two different sources of information when interpreting a sentence like this.
Semantic heuristics about typical agents (e.g., dogs) and patients (e.g., people) of biting events.
The syntactic structure of the sentence.
For active, implausible sentences (“The man bit the dog”), the implausibility is easier to identify—perhaps because active sentences are easier to parse. But for passive, implausible sentences (“The dog was bitten by the man”), those semantic heuristics seem to win out at least some of the time, almost as if people are relying on a kind of “bag-of-words” model.
Of course, most of the time that simple heuristic would work just fine: it would be good-enough. And among the other demands of rapid-fire conversation, “good-enough” may be the best we can manage.
Cognition is resource-constrained
Few things in life are optimal. Human cognition, after all, faces a number of constraints, and optimization is expensive. We don’t have infinite time or resources with which to make a decision, which means we’ve got to settle for “good-enough”. This is true for communication, and it’s also true for decision-making more generally.
Importantly, the fact that we often rely on “cheap tricks” isn’t necessarily evidence of irrationality. As I’ve written before, it can also be interpreted as evidence that we’ve evolved to solve problems under a set of constraints:
Moreover, when one considers the fact that decisions are rarely made with perfect knowledge of the world, but are instead made under conditions of considerable uncertainty, some of these decision-making shortcuts start to make a lot more sense. In recent years, probabilistic models of cognition that emphasize the role of uncertainty have gotten a lot more popular for precisely this reason. That’s largely the insight behind bounded rationality: people make decisions with finite time and finite resources, and because of that, we opt for satisfactory outcomes—often using cheap cognitive tricks—rather than optimal ones. Under certain experimental conditions, these cheap tricks lead to apparently irrational behavior.
In this light, good-enough comprehension can be seen as a subset of a more general case whereby humans use heuristics or “cheap tricks” to solve problems. In a 2007 review paper on good-enough (GE) comprehension, the authors (Ferreira & Patson, 2007) write:
On this view, then, GE representations arise because the system makes use of a set of heuristics that allow it to do the least amount of work necessary to arrive at a meaning for a sentence.
If good-enough representations are usually good-enough, why do extra work when we don’t have to?
Cheap tricks and large language models
As readers of this newsletter will know, I spend a lot of time writing about large language models (LLMs). The original impetus for this post, in fact, was that LLMs are often accused of using “cheap tricks” that give the illusion of understanding, despite LLMs not truly understanding language. Sometimes LLMs are even compared to Clever Hans, a horse that was trained to perform arithmetic but which was later discovered to rely on spurious cues—like the unconscious behavior of his trainer.
To be clear, I think this is an important criticism to take seriously, and it relates to the question of what it means to display impressive performance on a benchmark or task. For example, do LLMs really have a “Theory of Mind”, or are they relying on low-level heuristics or shortcuts to solve tasks that assess Theory of Mind—a kind of “clever LLM” effect? Figuring this out is critical for determining which capacities LLMs have or don’t have, and how those capacities are actually implemented at a mechanistic level.
At the same time, I think it’s important to be clear-eyed about the fact that humans also rely on cheap tricks. And I don’t mean that in a deflationary way—I think it’s really remarkable how all biological organisms, including humans, have evolved to make do in a harsh, constantly changing environment, and that includes the heuristics we use to get by. But when people criticize LLMs for using shortcuts, there’s typically an implicit (and sometimes explicit) contrast being drawn with what humans do (e.g., “LLMs aren’t really reasoning to solve this task, they’re using shortcuts [whereas humans clearly are reasoning]”). If we’re going to make this contrast, we should point to empirical evidence that humans really are doing something different.
Related posts:
If you’re feeling good about the fact that you did notice an incongruity, consider the fact that you were warned ahead of time!